What is Robust Analytics?
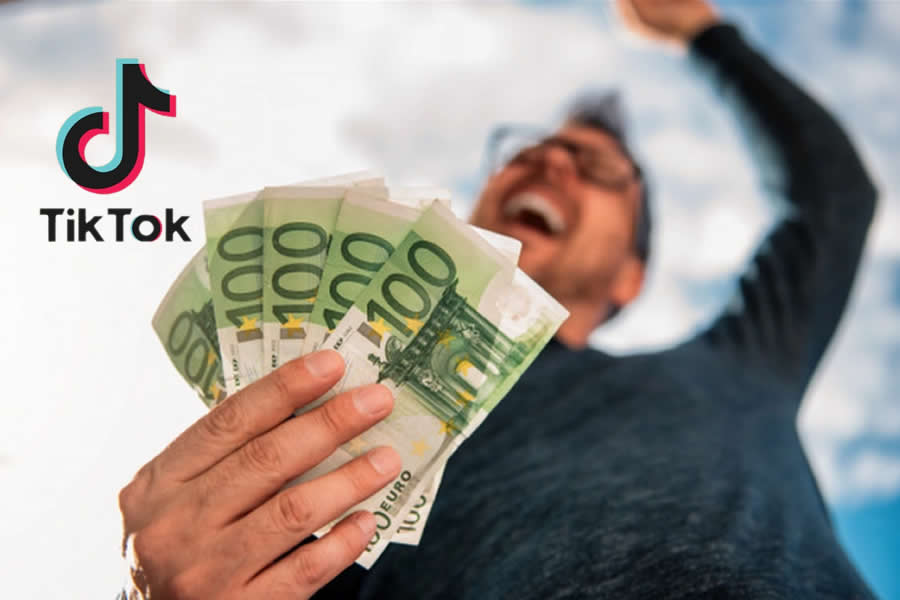
Robust analytics refers to analytical methods and techniques that are designed to be reliable and effective in a variety of conditions, even when data is noisy, incomplete, or subject to change. Unlike standard analytics, which might focus on specific or ideal conditions, robust analytics ensures that the insights derived are accurate and actionable, regardless of the challenges posed by the data.
Why Robust Analytics Matter
Data Quality and Consistency
In the real world, data is often imperfect. It may be incomplete, contain errors, or be subject to frequent changes. Robust analytics is designed to handle these imperfections effectively. For instance, techniques such as robust regression can provide reliable results even when there are outliers in the data, which would otherwise skew the results of more traditional methods.
Adaptability to Change
Markets and business environments are constantly evolving. Robust analytics allows businesses to adapt to these changes by using methods that remain effective even as the underlying data or conditions shift. This adaptability is crucial for maintaining accuracy and relevance in decision-making processes.
Resilience to Noise
Real-world data is often noisy, meaning it contains a lot of random variation that can obscure the true signal. Robust analytics employs methods that can filter out this noise, providing clearer insights and more reliable forecasts.
Key Techniques in Robust Analytics
1. Robust Regression
Robust regression techniques, such as Huber regression or Least Absolute Deviations (LAD) regression, are designed to reduce the impact of outliers on the results. Unlike ordinary least squares regression, which can be heavily influenced by extreme values, robust regression techniques minimize the effect of these outliers, leading to more reliable and stable models.
2. Bootstrapping
Bootstrapping is a resampling technique used to estimate the distribution of a statistic by repeatedly sampling with replacement from the data. This method helps in assessing the variability of an estimate and can be particularly useful when dealing with small sample sizes or uncertain data.
3. Robust Optimization
Robust optimization involves creating models that are less sensitive to uncertainties and variations in the data. By accounting for these uncertainties during the optimization process, businesses can develop solutions that are more reliable and perform well under a range of conditions.
4. Sensitivity Analysis
Sensitivity analysis examines how the output of a model responds to changes in input variables. By analyzing different scenarios and input variations, businesses can understand the robustness of their models and make more informed decisions based on how sensitive their results are to changes.
Applications of Robust Analytics
1. Financial Forecasting
In finance, robust analytics is crucial for creating reliable forecasts and managing risk. Financial models must account for market volatility and economic changes, and robust techniques help ensure that predictions remain accurate despite these uncertainties.
2. Marketing and Customer Insights
Marketing strategies often rely on customer data that can be noisy and variable. Robust analytics helps in deriving actionable insights from this data, allowing businesses to create targeted campaigns and improve customer engagement.
3. Supply Chain Management
Supply chains are complex and subject to many variables, including demand fluctuations and supply disruptions. Robust analytics enables companies to optimize their supply chains by creating models that can handle these uncertainties effectively.
4. Healthcare
In healthcare, robust analytics can improve patient outcomes by analyzing data from various sources, including electronic health records and clinical trials. By using robust methods, healthcare providers can make more accurate diagnoses and treatment recommendations.
Implementing Robust Analytics
1. Data Preparation
Effective robust analytics starts with high-quality data preparation. This includes cleaning the data, handling missing values, and dealing with outliers. Proper data preparation ensures that the analytical methods used are as effective as possible.
2. Choosing the Right Tools
There are various tools and software available for performing robust analytics. Choosing the right tools depends on the specific needs of the business and the type of data being analyzed. Common tools include statistical software like R and Python libraries such as Statsmodels and Scikit-learn.
3. Continuous Monitoring and Adjustment
Robust analytics is not a one-time process. Continuous monitoring of the data and models is necessary to ensure that they remain effective as conditions change. This involves regularly updating models, recalibrating parameters, and adjusting techniques as needed.
4. Training and Expertise
To effectively implement robust analytics, businesses need skilled personnel who understand both the technical aspects and the practical applications of these techniques. Investing in training and expertise is crucial for maximizing the benefits of robust analytics.
Conclusion
In summary, robust analytics is a critical approach for ensuring that analytical methods remain reliable and effective under various conditions. By employing techniques that handle data imperfections, adapt to changes, and filter out noise, businesses can derive more accurate insights and make better-informed decisions. Whether in finance, marketing, supply chain management, or healthcare, robust analytics plays a pivotal role in navigating the complexities of the data-driven world.
Embracing robust analytics means investing in methods and tools that provide resilience and reliability in the face of uncertainty. As data continues to be a cornerstone of decision-making, understanding and implementing robust analytics will be key to staying ahead in a competitive landscape.
Top Comments
No Comments Yet